This Benchmark is meant for research purposes by providing a pipeline to get scenarios usable to compare it with other state-of-the-art algorithms and datasets. The scenarios are based on the data provided by DeepScenario via drones from different location mostly in Germany. More details to our pipeline.
Munich Tal in crowded spaces
Background
Prediction and planning benchmarks are integral to the advancement of autonomous driving systems, serving as critical tools for assessing the efficacy of various approaches and facilitating their comparative analysis. Over recent years, the research community has increasingly turned its attention to complex urban scenarios, leading to the development and publication of numerous autonomous driving datasets designed to address these challenges. Notable examples include CommonRoad (2017), nuScenes (2019), Interaction (2019), nuPlan (2021), Waymo Open Motion (2021), and the Argoverse series (2019, 2021).
While the diversity of data in existing autonomous driving datasets is commendable, there remains a notable gap in capturing high-density interactions among road users. This limitation is critical, as it affects the ability of autonomous systems to effectively navigate and respond in densely populated urban environments.
One reason for this gap is that the majority of available datasets predominantly focus on environments in America and Asia, where road densities are approximately 36.02km/100km² and 68km/100km², respectively. This is in contrast to Germany, which features one of the highest road densities globally, approximately 180.51km/100km². Even in rural areas interaction between traffic participants is more pronounced, and visible.
And in contrast to many other parts of the world, European cities exhibit a smooth integration between different types of traffic participants, such as vehicles, and vulnerable road users (VRUs). This integration is facilitated also by traffic rules, and infrastructure that are tailored to enhance cooperation, and safety among all road users.
This discrepancy highlights a significant variation in the environmental complexity that datasets cover, suggesting an opportunity to expand dataset diversity to include regions with higher road densities like Germany. Such an expansion could potentially enhance the robustness, and applicability of autonomous driving technologies, ensuring their adaptability to a wider range of global, more complex traffic conditions, and infrastructural densities.
In collaboration with our industrial partner, DeepScenario, we created the “DeepUrban (V1)”—dataset to enhance benchmarking of trajectory prediction, and planning methods with a focus on dense urban settings with a heavy interaction between road users. DeepUrban is distinguished by its rich collection of relevant 3D traffic objects, extracted from high-resolution images captured by drones (cf. Figure below). The dataset is further enriched with comprehensive map, and scene information to support advanced modeling, and simulation tasks.
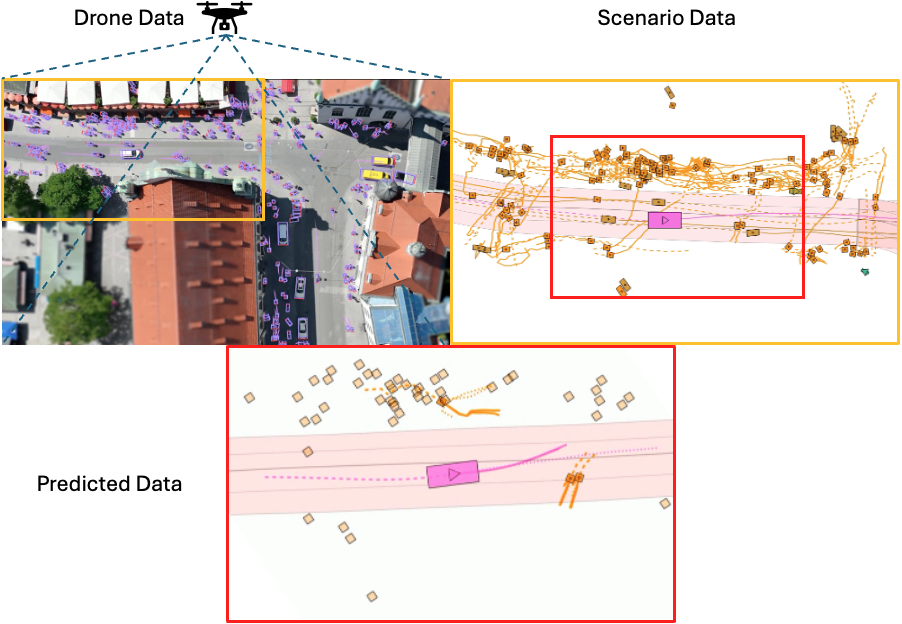
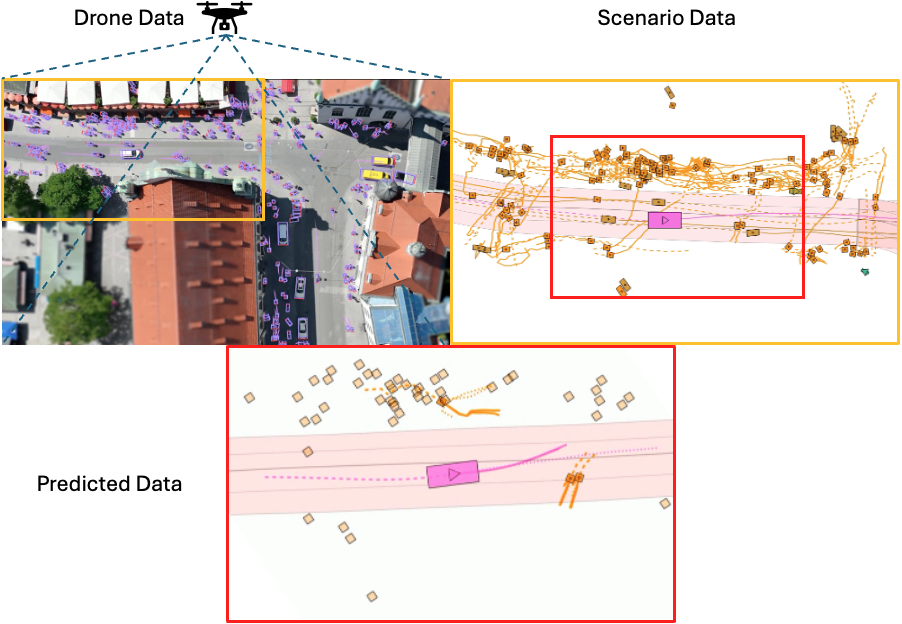
Acknowledgement
This work is a result of the joint research project STADT:up (19A22006N). The project is supported by the German Federal Ministry for Economic Affairs and Climate Action (BMWK), based on a decision of the German Bundestag. The author is solely responsible for the content of this publication.
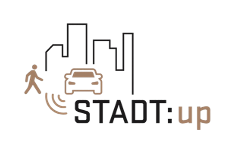
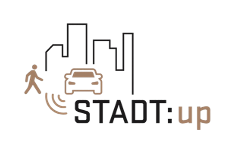
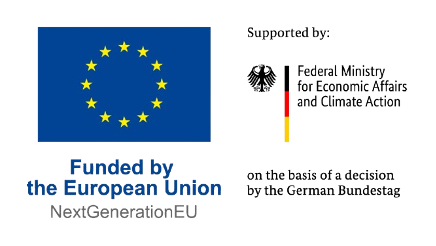
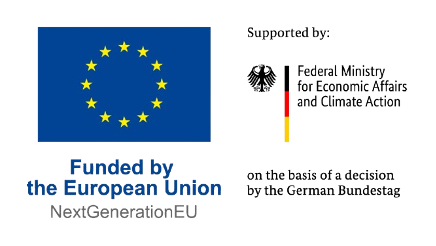