Walk-the-Talk addresses limitations in existing motion-language data and allows an efficient domain transfer to bridge the reality-gap in autonomous driving simulations. Generated motions by Walk-the-Talk model can be retargeted into a CARLA simulator to produce photo-realistic simulations of various pedestrian behaviours and scenarios.
Real-Time Inference
Walk-the-Talk generates 3D human motion in autonomous driving context in under 3 seconds on average!
Autonomous Driving use case
Our focus is to introduce a framework to improve autonomous driving capabilities as a whole with more realistic simulations. In the figure below we show rendered examples of various scenes in CARLA. The biggest failures of even the SOTA methods are in the edge cases and rare scenarios that are only present in the long-tail distribution of existing data. Using natural language allows us to easily vary the motions and behaviours of the agents and adapt it to a specific scenario. However, there exists a huge gap in available data structures that can facilitate these simulations. Walk-the-Talk is a first step in closing these domain and reality gaps as well as providing a pathway to enhance traditional autonomous driving simulators.
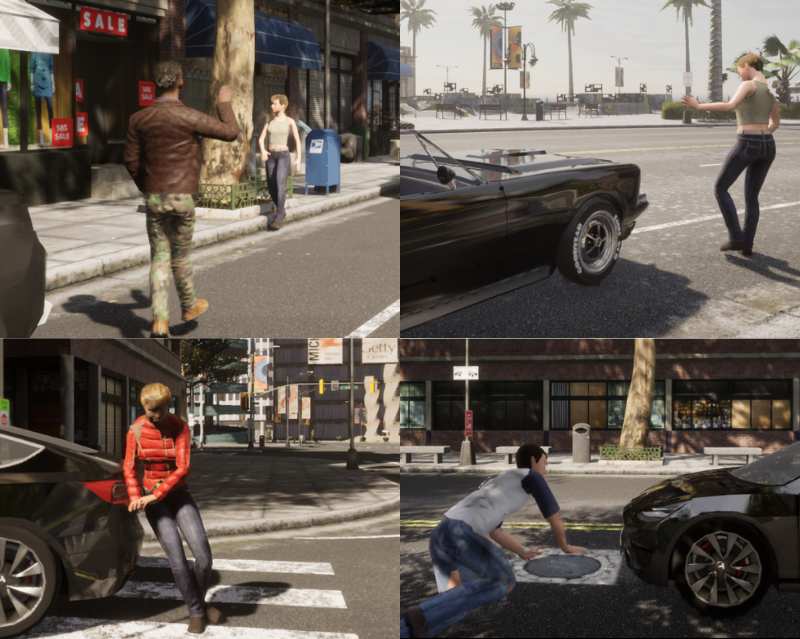
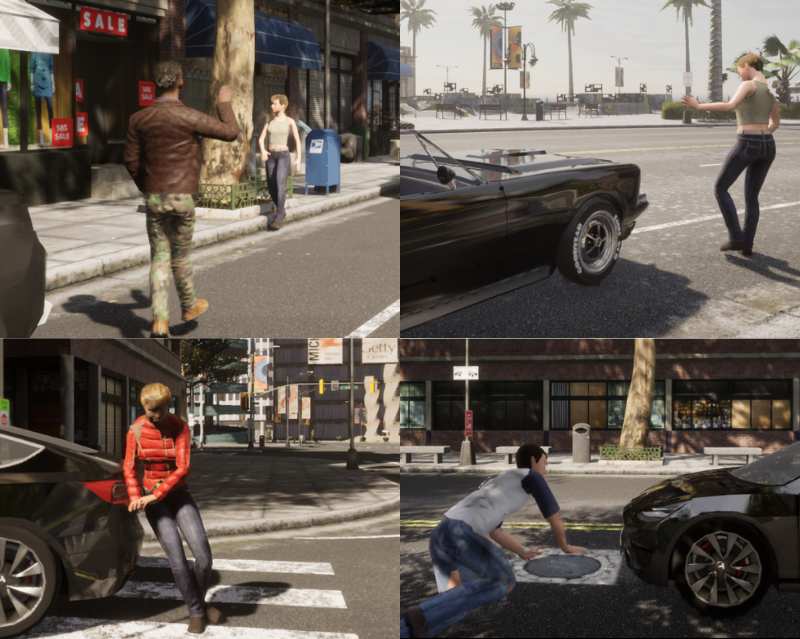
Cite as
@INPROCEEDINGS{10588860,
author={Ramesh, Mohan and Flohr, Fabian B.},
booktitle={2024 IEEE Intelligent Vehicles Symposium (IV)},
title={Walk-the-Talk: LLM driven pedestrian motion generation},
year={2024},
volume={},
number={},
pages={3057-3062},
keywords={Legged locomotion;Training;Pedestrians;Generative AI;Large language models;Semantics;Motion capture},
doi={10.1109/IV55156.2024.10588860}}
}
Acknowledgement
This work is a result of the joint research project STADT:up (19A22006N). The project is supported by the German Federal Ministry for Economic Affairs and Climate Action (BMWK), based on a decision of the German Bundestag. The author is solely responsible for the content of this publication.
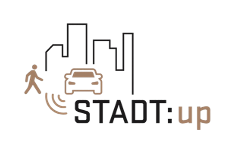
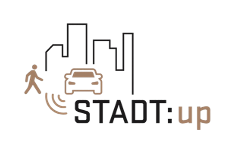
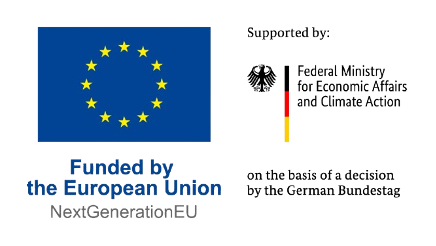
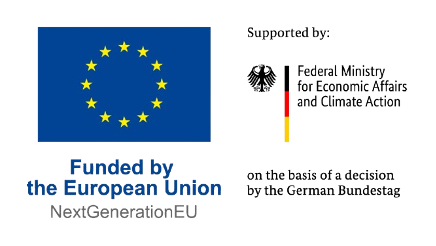