The Intelligent Vehicles Lab (founded in 2023) at the Munich University of Applied Sciences is dedicated to developing intelligent and safe transportation systems. One of their primary research focuses is on how to handle vulnerable road users (VRUs) among other traffic agents in the context of autonomous driving. The lab’s work includes developing algorithms and software that can detect, parse, track, and predict the behavior of traffic agents in complex urban environments leading to a robust ego-trajectory planning. The lab collaborates with industry partners to test their solutions in real-world settings, with the aim of advancing the state of the art in autonomous driving technology.
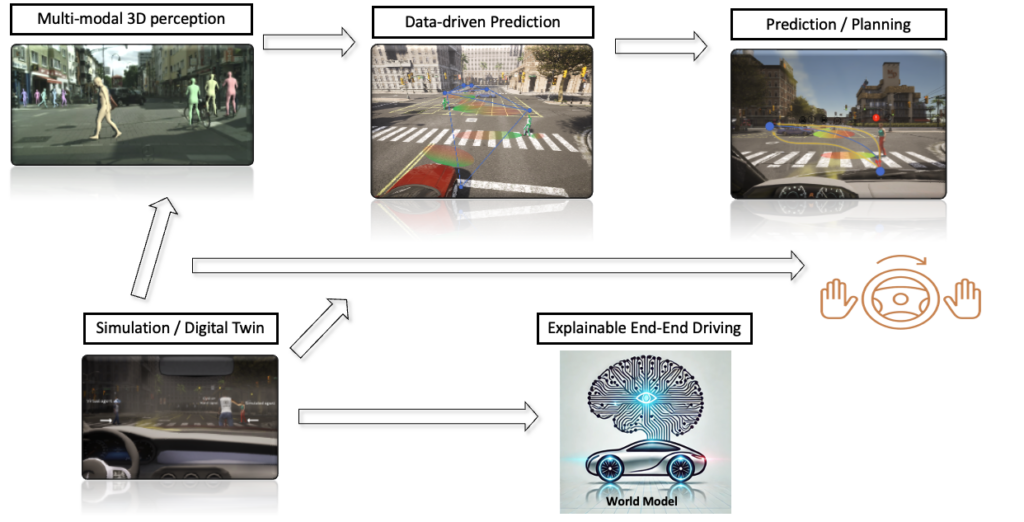
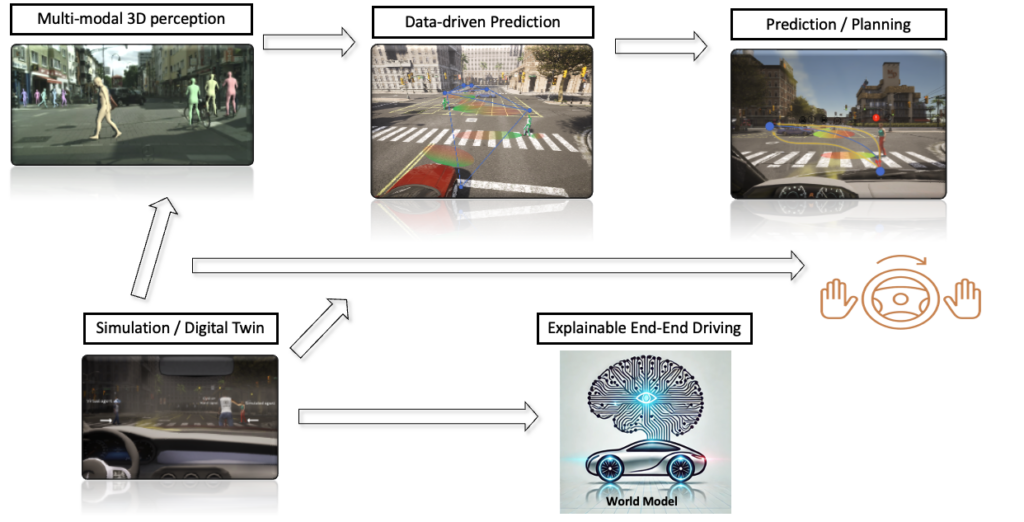
Why Autonomous Driving?
Autonomous driving refers to the ability of a vehicle to operate without human intervention. This is achieved through the use of advanced sensors, software, and other technologies that allow the vehicle to perceive and interpret its surroundings, make decisions, and take actions accordingly. Autonomous driving has the potential to significantly reduce traffic accidents, increase mobility for those who cannot drive, and reduce congestion and emissions. It also has the potential to transform transportation systems and improve the quality of life for people worldwide.
The role of the VRU
Vulnerable road users (VRUs) such as pedestrians, cyclists, and scooter riders play a critical role in urban automated driving. Since these users are more exposed and less protected than occupants of cars, they are at a higher risk of injury in accidents. Therefore, autonomous vehicles must be able to detect and respond to the presence and behavior of VRUs, such as predicting their movements and adjusting their own speed and trajectory accordingly. Ensuring the safety and mobility of VRUs is essential for the successful implementation of autonomous driving in urban environments.
A more detailed view
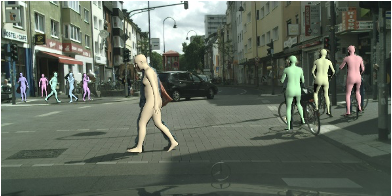
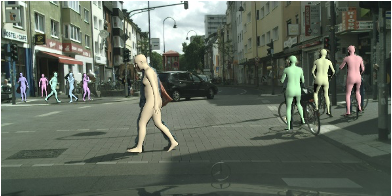
Perception & Pose estimation
An accurate perception and pose estimation are critical aspects of this safety improvement, as they enable vehicles to detect, parse and track vulnerable road users accurately. Challenges such as occlusions, varying lighting conditions, and different weather conditions must be considered when developing perception and pose estimation models for vulnerable road users. Furthermore, an accurate pose estimation enable up-stream tasks like intention and gesture recognition.
Lidar-Camera Sensor Fusion
Sensor fusion provides a more comprehensive understanding of the environment and enables more accurate detection and tracking of road users. Lidar sensors provide accurate 3D point cloud data, while cameras provide high-resolution 2D images. While an object can be correctly localized with Lidar, fine-grained features can be extracted from the camera image. Combining these two types of data provides a more comprehensive understanding of the environment and enables more accurate detection and parsing of objects.
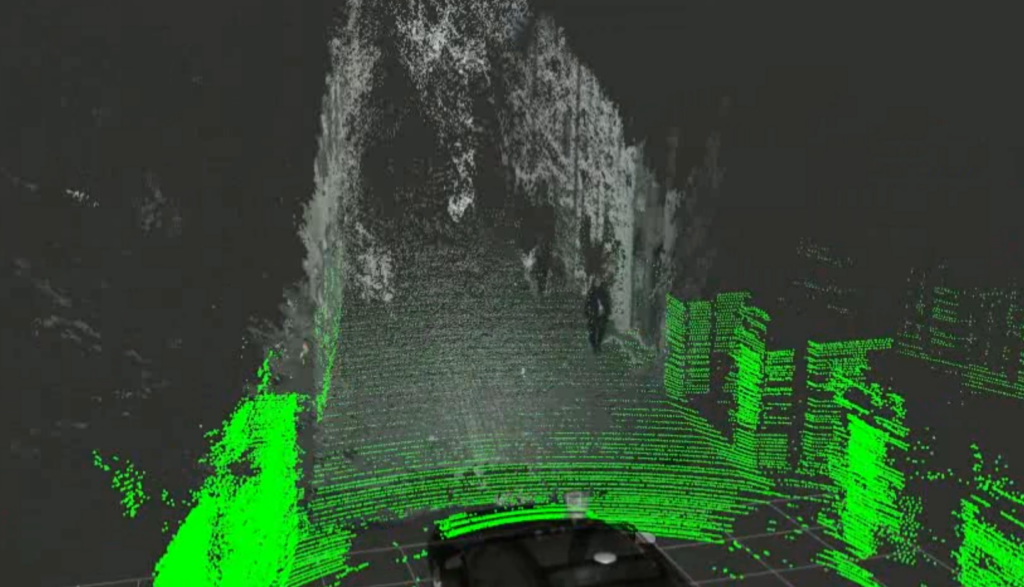
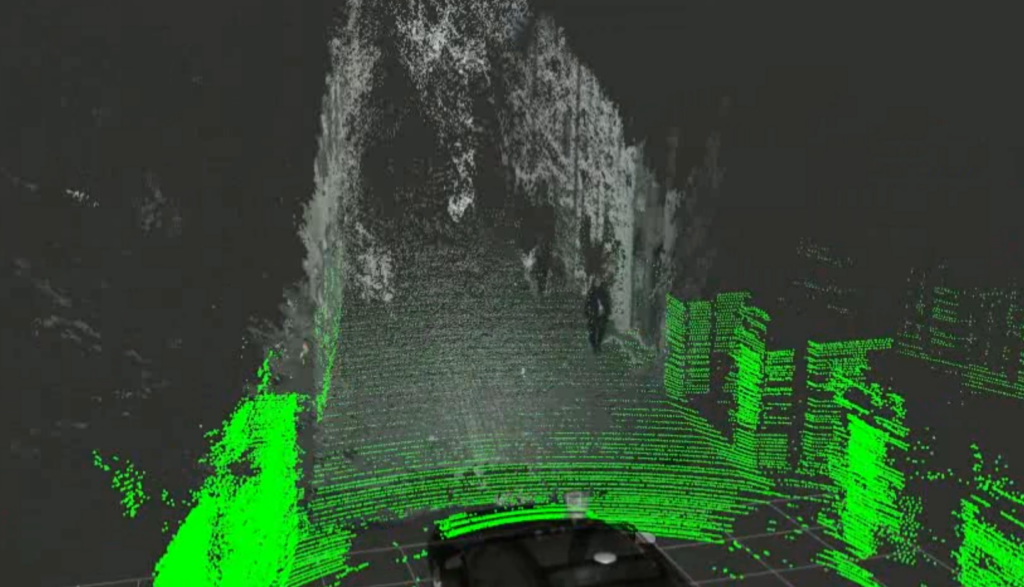
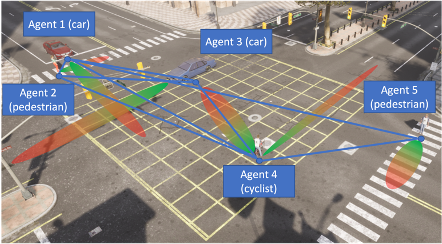
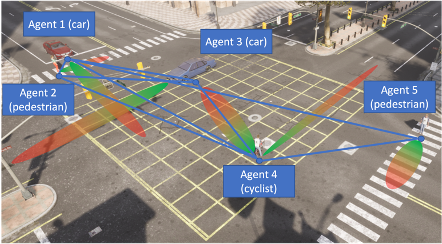
Multi-modal prediction
Data-driven, multi-modal prediction techniques are used to account for the multiple possible outcomes of road users’ movements. These techniques involve modeling the different potential outcomes of a road user’s actions based on an accurate perception and assigning probabilities to each outcome based on the available data. Uncertainty estimation is critical for assessing the reliability of the models and predictions developed for vulnerable road users.
End-End Concepts
To effectively plan for autonomous vehicles, it is essential to understand the intention and behaviors of the traffic agents in different scenarios. End-End concepts consider the entire functional chain (perception, prediction and planning) and implicitely model the interaction of AVs with the environment and other road users. End-End approaches enables autonomous vehicles to make better decisions, anticipate potential hazards, and respond appropriately to unexpected situations.
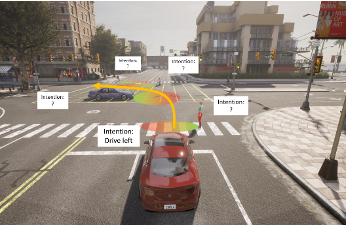
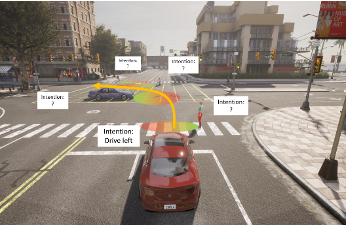
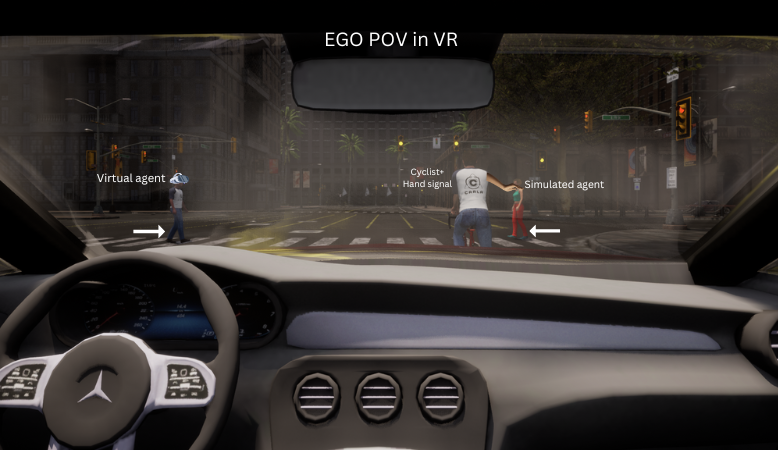
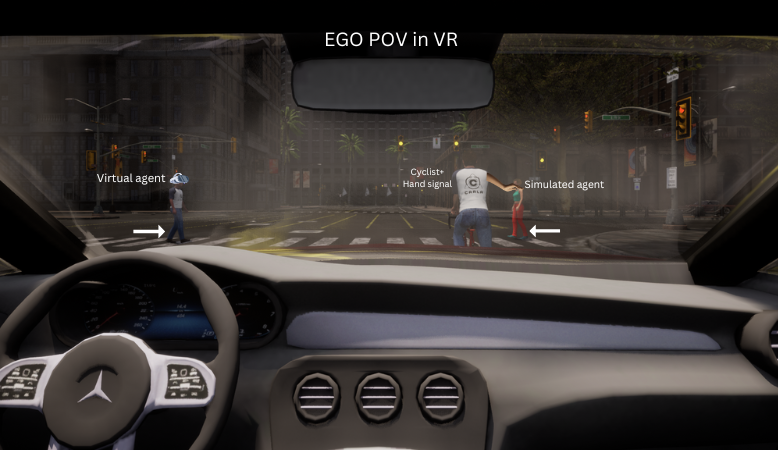
Simulation & Digital Twin
A simulation of rare and critical scenarios is essential for understanding the behavior of vulnerable road users. We make use of virtual reality (VR) technology and motion capture for creating these simulations and analyzing the results. The development of digital twins, which are digital replicas of real-world traffic environments, has the potential to revolutionize the understanding of vulnerable road users’ behavior. Digital twins can provide a highly accurate representation of the traffic environment, enabling more realistic simulations of rare and critical scenarios.